Which machine learning models are commonly used for analyzing cryptocurrency market data?

What are some commonly used machine learning models for analyzing data in the cryptocurrency market?
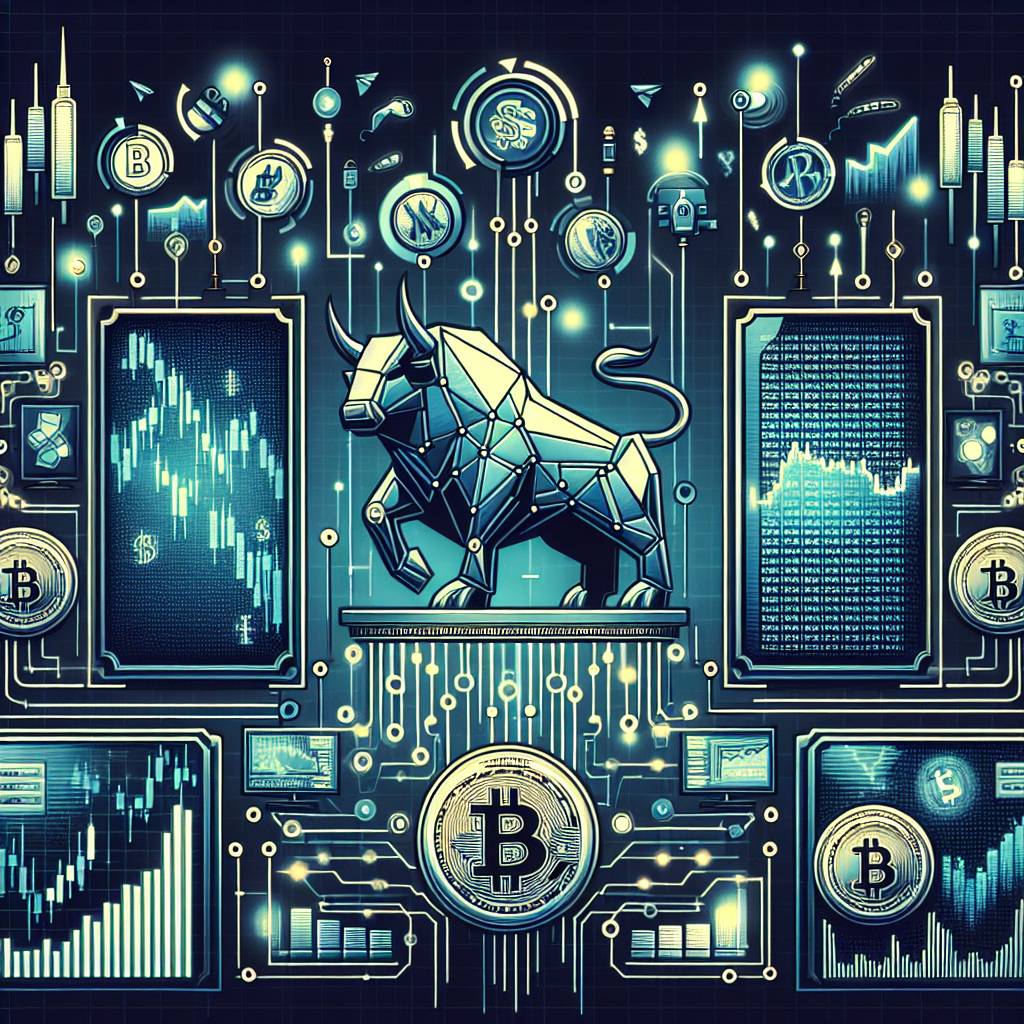
4 answers
- There are several machine learning models that are commonly used for analyzing data in the cryptocurrency market. One popular model is the Long Short-Term Memory (LSTM) network, which is a type of recurrent neural network (RNN). LSTM networks are known for their ability to capture long-term dependencies in sequential data, making them suitable for analyzing time series data such as cryptocurrency prices. Another commonly used model is the Random Forest algorithm, which is an ensemble learning method that combines multiple decision trees to make predictions. Random Forests are often used for feature selection and classification tasks in the cryptocurrency market. Additionally, Support Vector Machines (SVM) and Gradient Boosting Machines (GBM) are also popular machine learning models used for analyzing cryptocurrency market data. SVMs are effective for both classification and regression tasks, while GBMs are known for their ability to handle large datasets and capture complex relationships between variables.
Dec 16, 2021 · 3 years ago
- When it comes to analyzing cryptocurrency market data using machine learning models, there are a few go-to options. One popular choice is the Long Short-Term Memory (LSTM) network, which is a type of recurrent neural network (RNN). LSTM networks are well-suited for analyzing time series data, such as cryptocurrency prices, as they can capture long-term dependencies. Another commonly used model is the Random Forest algorithm, which is an ensemble learning method that combines multiple decision trees. Random Forests are often used for feature selection and classification tasks in the cryptocurrency market. Support Vector Machines (SVM) and Gradient Boosting Machines (GBM) are also commonly employed for analyzing cryptocurrency market data. SVMs are versatile and can handle both classification and regression tasks, while GBMs excel at handling large datasets and capturing complex relationships between variables.
Dec 16, 2021 · 3 years ago
- When it comes to analyzing cryptocurrency market data using machine learning models, there are a few options that are commonly used. One popular choice is the Long Short-Term Memory (LSTM) network, which is a type of recurrent neural network (RNN). LSTM networks are great for analyzing time series data, like cryptocurrency prices, because they can capture long-term dependencies. Another commonly used model is the Random Forest algorithm, which is an ensemble learning method that combines multiple decision trees. Random Forests are often used for feature selection and classification tasks in the cryptocurrency market. Support Vector Machines (SVM) and Gradient Boosting Machines (GBM) are also commonly employed for analyzing cryptocurrency market data. SVMs are versatile and can handle both classification and regression tasks, while GBMs excel at handling large datasets and capturing complex relationships between variables.
Dec 16, 2021 · 3 years ago
- BYDFi, a leading digital asset exchange, has found that machine learning models play a crucial role in analyzing cryptocurrency market data. One commonly used model is the Long Short-Term Memory (LSTM) network, which is a type of recurrent neural network (RNN). LSTM networks are particularly effective for analyzing time series data, such as cryptocurrency prices, as they can capture long-term dependencies. Another popular model is the Random Forest algorithm, which is an ensemble learning method that combines multiple decision trees. Random Forests are often used for feature selection and classification tasks in the cryptocurrency market. Support Vector Machines (SVM) and Gradient Boosting Machines (GBM) are also commonly employed for analyzing cryptocurrency market data. SVMs are known for their versatility in handling both classification and regression tasks, while GBMs excel at handling large datasets and capturing complex relationships between variables.
Dec 16, 2021 · 3 years ago
Related Tags
Hot Questions
- 97
What are the best digital currencies to invest in right now?
- 91
What are the best practices for reporting cryptocurrency on my taxes?
- 81
What is the future of blockchain technology?
- 76
What are the tax implications of using cryptocurrency?
- 69
How can I buy Bitcoin with a credit card?
- 68
How can I minimize my tax liability when dealing with cryptocurrencies?
- 52
How can I protect my digital assets from hackers?
- 27
How does cryptocurrency affect my tax return?